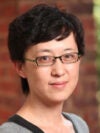
Qi, Yanjun
Primary Appointment
Computer Science
Contact Information
we have devoted our research efforts on both the improvement of machine learning technologies and the advancement of biomedical research.
Email: yq2h@virginia.edu
Research Description
UVA Machine Learning and Bioinformatics" group's research has been focused on developing and applying machine-learning techniques on important challenges in biomedicine