Jiaxing (Joy) Qiu, MS, PhD
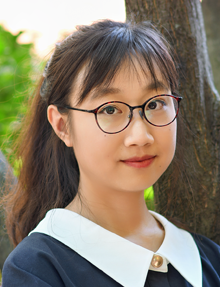
Jiaxing (Joy) Qiu, PhD Candidate
CAMA Data Scientist
Jiaxing Qiu has been a data scientist for CAMA since 2021. She earned her B.S. in Information and Computing Science from the School of Mathematical Sciences at Soochow University, China mainland. She is a current Ph.D. candidate in Data Science at the University of Virginia, where she also earned a MS in Data Science.
Her research focuses on statistical analysis and predictive modeling, utilizing multivariate time series data, which combines physiological measurements from bedside cardiovascular monitors with clinical and laboratory measurements from patient health records. She has collaborated with Dr. Randall Moorman, Dr. Chris Moore, and Dr. Rich Nguyen on detecting pathophysiological responses to bloodstream infections in critically ill transplant recipients compared to non-transplant recipients, resulting in a publication in Clinical Infectious Diseases. Jiaxing also works with Dr. Randall Moorman and Dr. Douglas Lake on predicting unfavorable cardiorespiratory outcomes in extremely preterm infants as part of the Pre-Vent study, contributing to multiple publications. Additionally, she collaborates with Dr. Brynne Sullivan and Dr. Karen Fairchild on predictive modeling and statistical analysis of various NICU patient conditions, such as early-onset sepsis, late-onset sepsis, and cerebral palsy, contributing to several publications in the Pre-Mo study.
Her current research emphasizes improving computational efficiency in predictive modeling through innovative statistical methods. She collaborates with Dr. Douglas Lake on the methodological publication “Fast Leave-One-Cluster-Out Cross-Validation Using Clustered Network Information Criteria (NICc),” with the resulting method expected to be widely used in future CAMA predictive modeling of repeatedly measured patient data. She also serves as a research consultant for Nihon Kohden Digital Health Solutions, Inc., working on collaborations to develop and validate predictive model implementations for real-time bedside monitors.