About
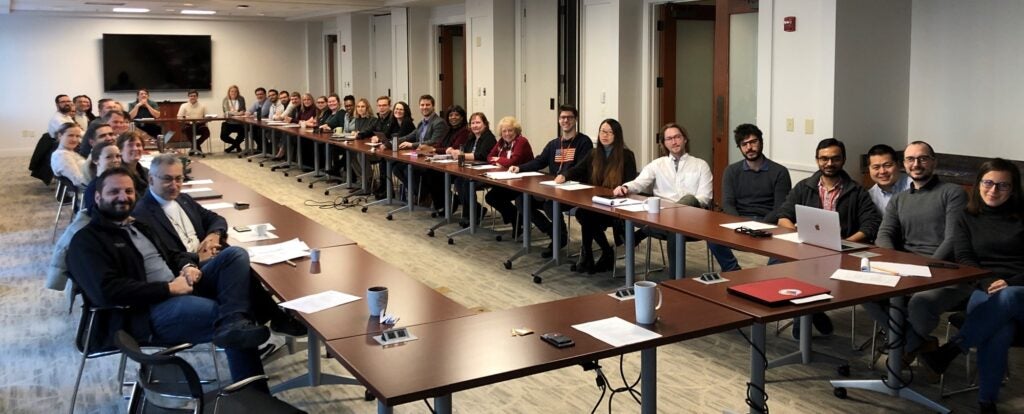
Center for Diabetes Technology (CDT) Team
History
Contact Us
For more information about the work being done at the Center for Diabetes Technology, please explore the website or
The Center for Diabetes Technology (CDT) was originally established in 2010 in association with the Departments of Psychiatry and Neurobehavioral Sciences Behavioral Sciences, Endocrinology, and the School of Engineering. In 2017, the CDT was formally established as an independent Center. The CDT has pursued a path to advance the use of technology, including analytics, to allow improved management, monitoring, and therapies for patients with Type 1 and Type 2 Diabetes.
Now, the CDT is a recognized world leader in the technological treatment of diabetes and a hub of an international research network of leading U.S. institutions (Harvard, Stanford, Yale, Mayo Clinic, Mt. Sinai School of Medicine) and worldwide groups in Denmark, Italy, France, Holland, and Argentina.
Building on the translational background in Type 1 diabetes, the CDT aims to grow the clinical and industrial translation of in silico augmented technologies to improve the management, monitoring, and therapies of diabetes for a larger population of people to include patients with Type 2 diabetes (T2D). This can be made possible through continued partnerships within UVA academics, expanded partnership with affiliate healthcare institutions, and the development of academic-industry relationships. Through industry affiliations, particularly those associated with T2D decision support systems and new medications, the CDT can contribute expert knowledge in technological therapy and modeling of T1D to further advance the treatment of diabetes.
Contact Us
For more information about the work being done at the Center for Diabetes Technology, please explore the website or
Diabetes Data Science
During 2017, a significant project was introduced at UVA under the direction of the CDT, entitled the Precision Individualized Medicine for Diabetes – The Virginia PrIMeD Project. In brief, the Virginia PrIMeD Project can be described as a framework built upon the research, clinical, educational, and technology‐transfer experience at UVA to create a unique and comprehensive process encompassing all aspects of T1D, from detection of genetic risk and markers of disease onset, through optimal maintenance and control of T1D via the artificial pancreas, to investment in a cure derived from future immunotherapeutic solutions. Building on the PrIMeD Project and utilizing the technology of several domestic and international trials involving decision support systems or artificial pancreas systems, the ultimate goal is to establish a new scientific discipline– Diabetes Data Science. This new scientific discipline will bridge genetic information, electronic health records, real-time monitoring of physiological and behavioral parameters, and automated control of diabetes. The immediate objective towards this goal is to create and pilot an innovative Diabetes Data Science paradigm – the Virtual Image of the Patient (VIP) – which will allow an in silico image of each patient to be built in a database, initialized with the individual’s genome, and then updated with physiologic and behavioral phenotypes. To achieve this goal, the CDT aspires to expand their research portfolio through collaboration with other UVA researchers across previously unrelated disciplines.
The Virtual DCCT Project
The Diabetes Control and Complications Trial (DCCT) was a 10-year controlled clinical trial conducted from 1982 to 1993 and published in the NEJM. Where 1,441 participants with Type 1 Diabetes were followed for an average 6.5 years. The purpose of the study was to compare intensive therapy (INT), aimed at achieving levels of glycemia as close to the nondiabetic range as safely possible, with conventional therapy (CON), which aimed to maintain safe asymptomatic glucose control. The Epidemiology of Diabetes Interventions and Complications (EDIC) observational follow-up determined the durability of the DCCT effects on the more-advanced stages of diabetes complications including cardiovascular disease (CVD). Together, the DCCT/EDIC defined A1c as a gold-standard marker of diabetes control and demonstrated the effectiveness of intensive therapy in reducing the long-term complications of T1D and improving the prospects for a healthy life span.
The Center for Diabetes Technology plans to replay this landmark study in computer simulation. To test that Continuous Glucose Monitoring (CGM) data can predict complications as well as A1c levels. This Virtual DCCT Project will give credibility to in silico augmented clinical trials and will expand our research into biosimulation.
Research Contributions
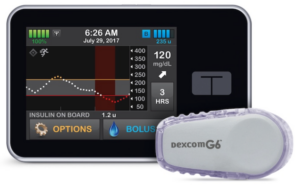
The artificial pancreas system with Control-IQ technology.
Over the years, the CDT has made many significant contributions in the management, monitoring, and therapies for patients with Type 1 Diabetes, including advanced analytics allowing individualized risk assessment, real-time prediction and prevention of acute events such as hypoglycemia. These advancements include the most comprehensive to date in silico model of the human metabolism – the only model accepted by the FDA as a substitute to animal trials in the testing of diabetes treatments, and the first portable Artificial Pancreas System. Translation of science, creation of intellectual property, and the development of academic-industry relationships are, and will continue to be, central to the Center moving forward.
Neural-Net Artificial Pancreas
Recently submitted to the Diabetes Technology & Therapeutics Journal (DTT) for publication, the Neural-Net Artificial Pancreas (NAP) is a new class of control algorithms. This type of algorithm is faster, lightweight, and can be pre-trained for the specific user. Meaning, the algorithm learns based on the user’s data and the data from others, also known as reinforcement learning or artificial intelligence (AI). This direction of algorithm will become central to our future research studies in collaboration with the UVA School of Data Science.